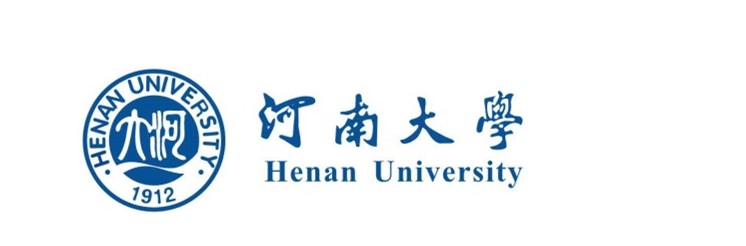
河南大学
2021年统计学前沿问题学术研讨会
暨博士后论坛
会议手册
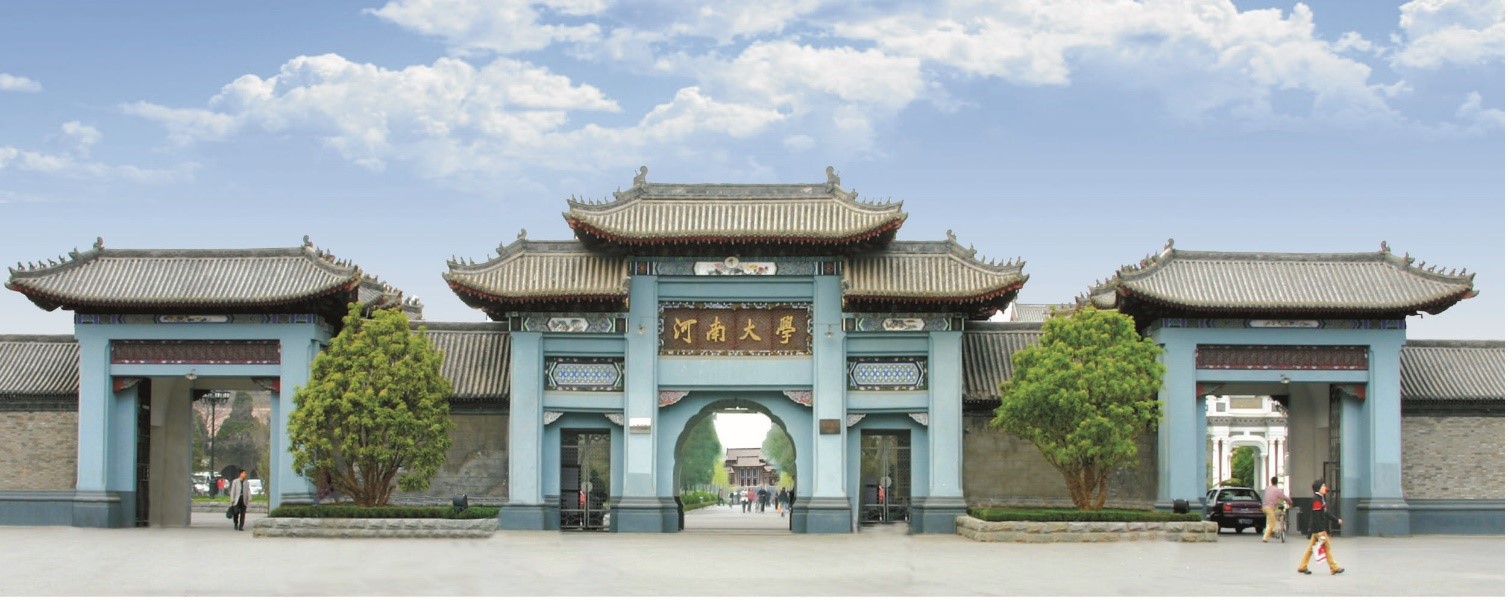
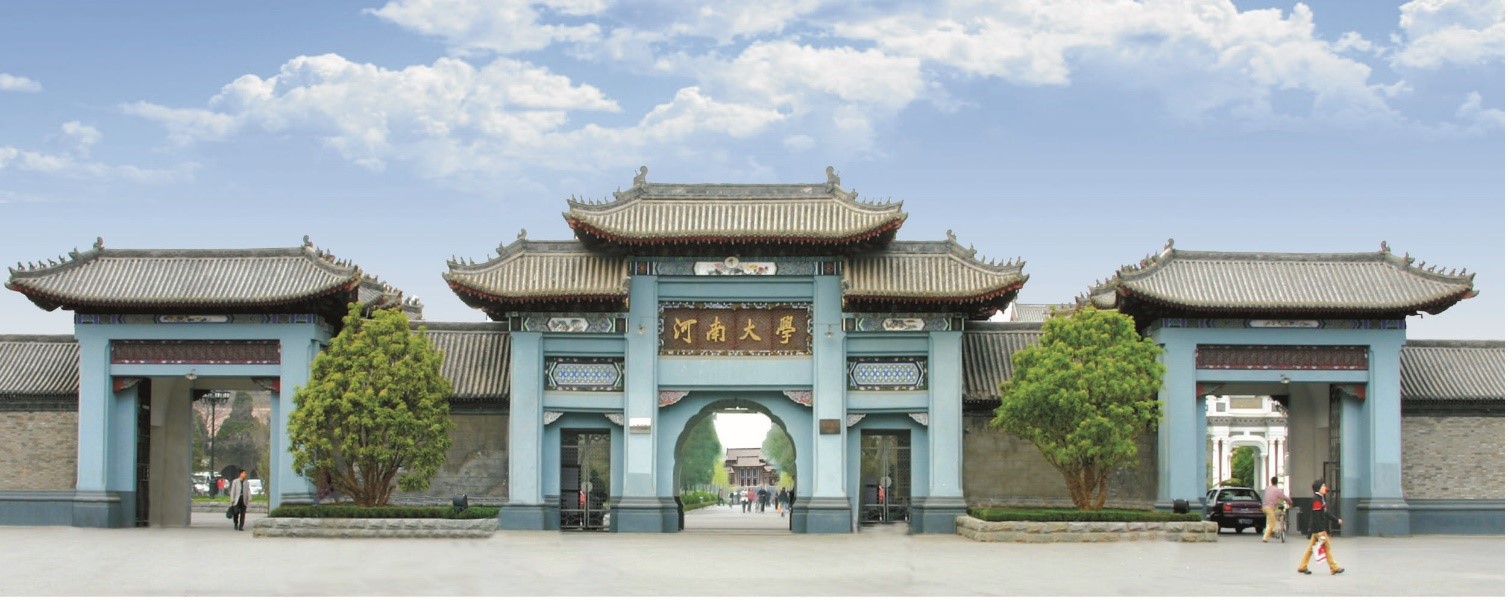
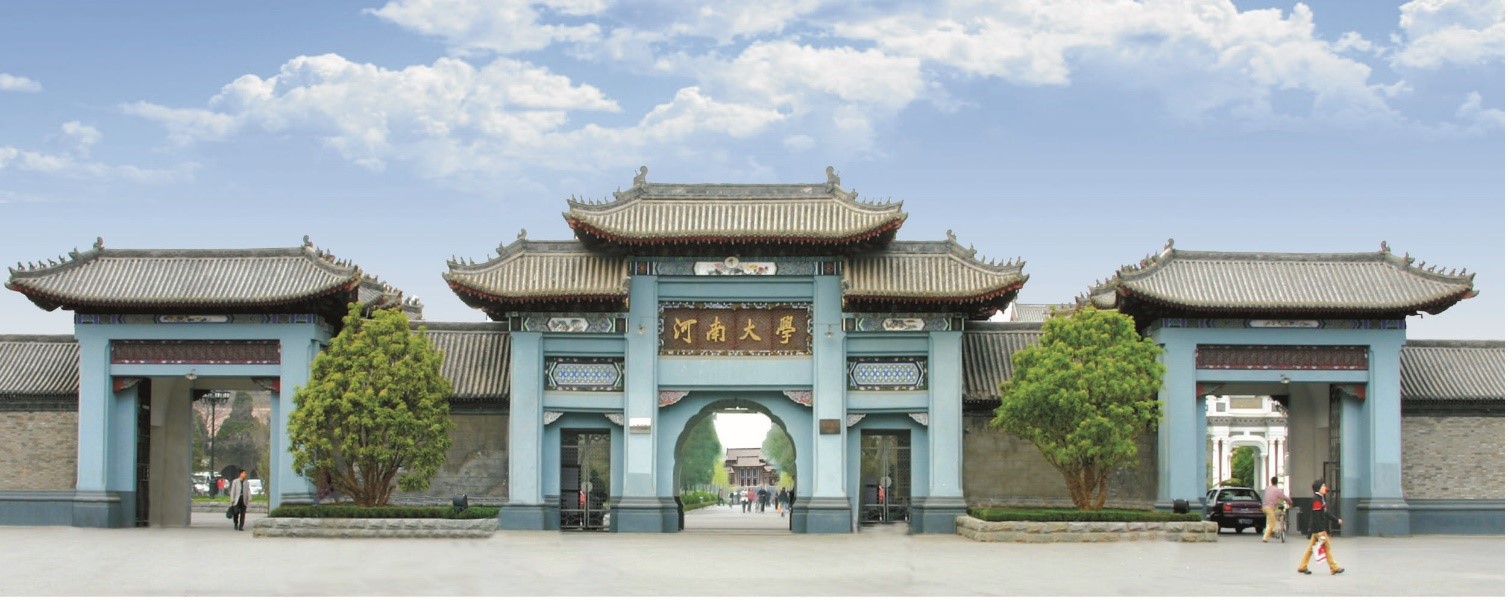
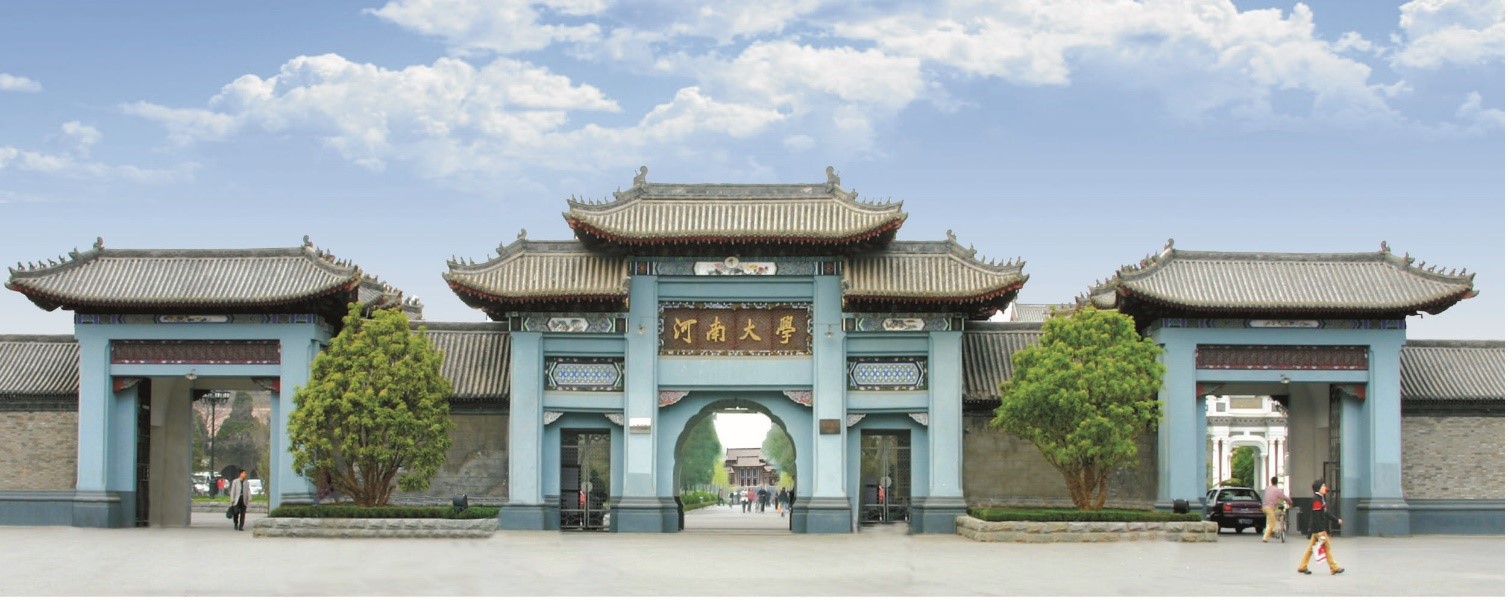
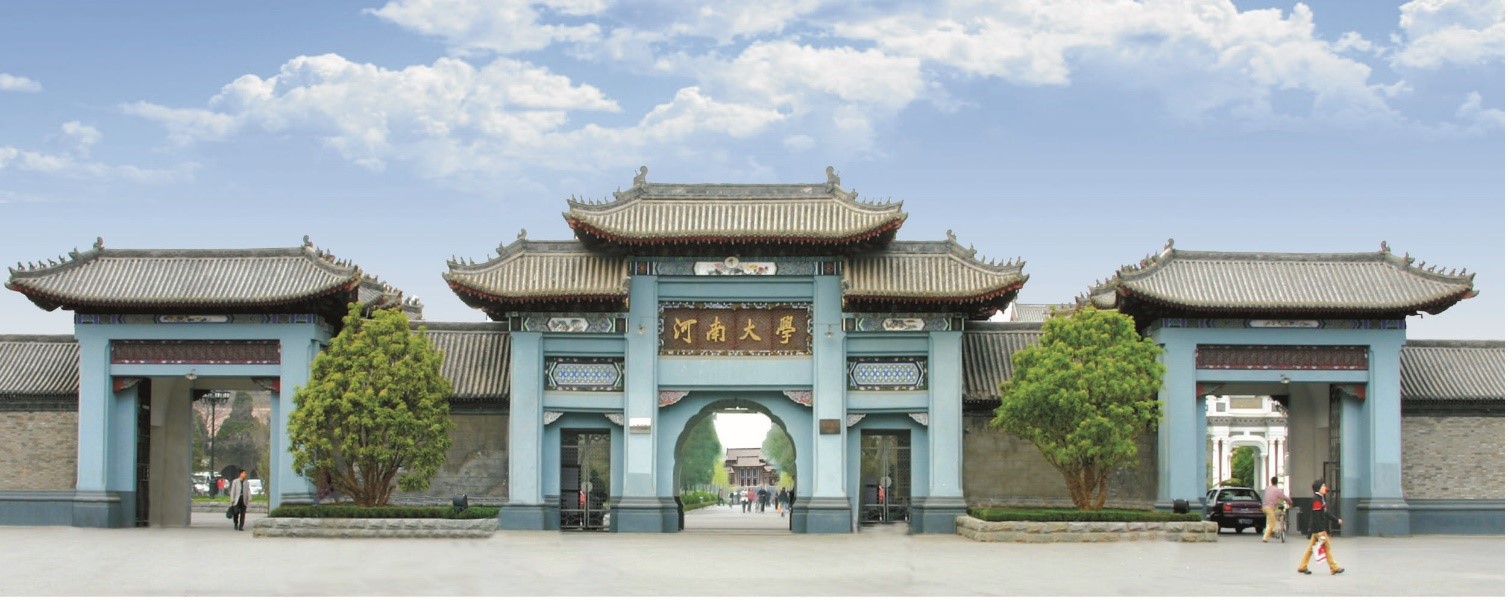
7月16日-7月18日
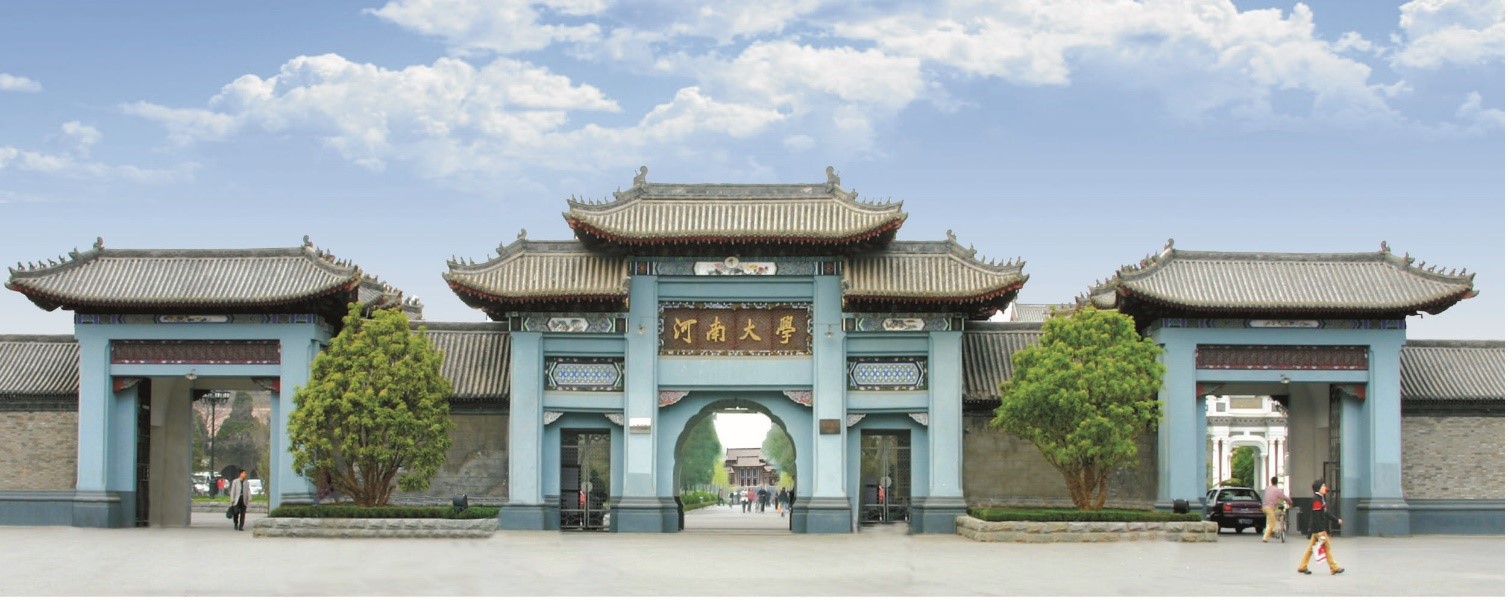
日程安排
2021年7月16日(星期五),全天报到 |
2021年7月17日(星期六)上午,腾讯会议ID: 895 7423 8582 线下会议地点:河南大学数学与统计学院 一楼报告厅 |
时间 |
报告人 |
报告题目 |
8:30-9:00 |
开幕式、合影留念 |
主持人: 解俊山(河南大学) |
主持人: 薛留根(北京工业大学) |
9:00-9:45 |
崔恒建 (首都师范大学) |
对抗学习中的稳健统计方法 |
9:45-10:30 |
钟威 (厦门大学) |
Multi-Kink Quantile Regression for Longitudinal Data with Application to Progesterone Data Analysis |
10:30-10:45 |
休息讨论 |
主持人:梁汉营(同济大学) |
10:45-11:30 |
林路 (山东大学) |
Unified Rules of Renewable Weighted Sums for Various Online Updating Estimations |
11:30-12:00 |
杨晓慧 (河南大学) |
Adaptive factorization rank selection-based NMF for high-dimensional data processing |
2021年7月17日(星期六)下午,腾讯会议ID: 895 7423 8582 线下会议地点:河南大学数学与统计学院 一楼报告厅 |
主持人: 庞善起(河南师范大学) |
14:00-14:45 |
童行伟 (北京师范大学) |
A weighted method for the exclusive hypothesis test with application to typhoon data |
14:45-15:30 |
李勇 (成都信息工程大学) |
智能大数据时代的统计学发展 |
15:30-15:45 |
休息讨论 |
主持人:解俊山(河南大学) |
15:45-16:30 |
刘党政 (中国科学技术大学) |
Non-Hermitian matrix-valued Brownian motion |
16:30-17:00 |
冯三营 (郑州大学) |
Estimation in functional single-index varying coefficient model |
17:00-17:30 |
伊梦茜 (对外经济贸易大学) |
Lassoing Eigenvalues |
2021年7月18日(星期天)上午,腾讯会议ID: 895 7423 8582 线下会议地点:河南大学数学与统计学院 一楼报告厅 |
主持人:张军舰(广西师范大学) |
8:30-9:15 |
刘民千 (南开大学) |
列正交强正交表的构造(线上) |
9:15-9:45 |
刘鹏飞 (江苏师范大学) |
Some applications of Median-of-Means method |
9:50-10:20 |
休息讨论 |
主持人:郑晨(河南大学) |
10:20-10:50 |
李哲源 (河南大学) |
L1正则下的自适应P-样条估计与节点自动选择(线上) |
10:50-11:20 |
刘中强 (河南理工大学) |
Subgroup-adaptive randomization for subgroup confirmation in clinical trials |
2021年7月18日(星期天)下午 |
自由交流、离会 |
题目与摘要
Title:对抗学习中的稳健统计方法
Speaker:崔恒建 (首都师范大学)
Abstract: 在机器学习中,经常出现两类特殊的异常样本:对抗样本和离群样本,这两类样本的存在,不仅会导致深度学习模型的性能大幅下降,还会存在很大的安全隐患。如何检测和识别这类在机器学习中出现的异常样本是人们极其感兴趣的问题。本演讲从该问题出发,介绍稳健统计思想和方法,包括稳健性、污染分布、崩溃点,影响函数等概念,并同时介绍MCD、T-型等实用稳健估计方法。进一步把这些稳健统计方法用到对抗学习等实例中去,结果表明稳健统计方法对提高机器学习及其数据处理的稳定性和有效性起着极其重要的作用。
Title:Multi-Kink Quantile Regression for Longitudinal Data with Application to Progesterone Data Analysis
Speaker:钟威 (厦门大学)
Abstract: Motivated by investigating the relationship between progesterone and the days in a menstrual cycle in a longitudinal study, we propose a multi-kink quantile regression model for longitudinal data analysis. It relaxes the linearity condition and assumes different regression forms in different regions of the domain of the threshold covariate. In this paper, we first propose a multi-kink quantile regression for longitudinal data. Two estimation procedures are proposed to estimate the regression coefficients and the kink points locations: one is a computationally efficient profile estimator under the working independence framework while the other one considers the within-subject correlations by using the unbiased generalized estimation equation approach. The selection consistency of the number of kink points and the asymptotic normality of two proposed estimators are established. Secondly, we construct a rank score test based on partial subgradients for the existence of kink effect in longitudinal studies. Both the null distribution and the local alternative distribution of the test statistic have been derived. Simulation studies show that the proposed methods have excellent finite sample performance. In the application to the longitudinal progesterone data, we identify two kink points in the progesterone curves over different quantiles and observe that the progesterone level remains stable before the day of ovulation, then increases quickly in five to six days after ovulation and then changes to stable again or even drops slightly.
Title: Unified Rules of Renewable Weighted Sums for Various Online Updating Estimations
Speaker: 林路(山东大学)
Abstract: We establish unified frameworks of renewable weighted sums (RWS) for various online updating estimations in the models with streaming data sets. The newly defined RWS lays the foundation of online updating likelihood, online updating loss function, online updating estimating equation and so on. The idea of RWS is intuitive and heuristic, and the algorithm is computationally simple. We choose nonparametric model as an exemplary setting. The RWS applies to various types of nonparametric estimators, which include but are not limited to nonparametric likelihood, quasi-likelihood and least squares. Furthermore, the method and the theory can be extended into the models with both parameter and nonparametric function. The estimation consistency and asymptotic normality of the proposed renewable estimator are established, and the oracle property is obtained. Moreover, these properties are always satisfied, without any constraint on the number of data batches, which means that the new method is adaptive to the situation where streaming data sets arrive perpetually. The behavior of the method is further illustrated by various numerical examples from simulation experiments and real data analysis.
Title: Adaptive factorization rank selection-based NMF for high-dimensional data processing
Speaker: 杨晓慧(河南大学)
Abstract: The nonnegative matrix factorization (NMF) has been widely used because it can accomplish both feature representation learning and dimension reduction. However, there are two critical and challenging issues affecting the performance of NMF models. One is the selection of matrix factorization rank, while most of the existing methods are based on experiments or experience. For tackling this issue, an adaptive and stable NMF model is constructed based on an adaptive factorization rank selection (AFRS) strategy, which skillfully and simply integrates a row constraint similar to the generalized elastic net. The other is the sensitivity to the initial value of the iteration, which seriously affects the result of matrix factorization. This issue is alleviated by complementing NMF and deep learning each other and avoiding complex network structure. The proposed NMF model is called deep AFRS-NMF model for short, and the corresponding optimization solution, convergence and stability are analyzed. Moreover, the statistical consistency is discussed between the rank obtained by the proposed model and the ideal rank. The performance of the proposed deep AFRS-NMF model is demonstrated by applying in genetic data based tumor recognition. Experiments show that the factorization rank obtained by the deep AFRS-NMF model is stable and superior to classical and state-of-the-art methods.
Title:Integrated Partition-Mallows Model and Its Inference for Rank Aggregation
Speaker:童行伟 (北京师范大学)
Abstract: Motivated by the testing of genetic pleiotropy, we discuss a general class of hypothesis testing, the exclusive hypothesis test (EHT). A hypothesis test is EHT if the null hypothesis can be divided into a set of exclusive sub-hypotheses, and a main difficulty for testing an EHT is the calculation of the p-value. To address this problem, we propose a weighted procedure and develop two methods, one likelihood-based and the other BIC-based, for determining the corresponding weights. Furthermore, we show that the BIC-based method can control the asymptotic type I error. We conduct an extensive simulation study of these two proposed methods, which suggests that they work well in practice. In particular, the new procedure is shown both theoretically and numerically to exhibit better performance than the existing two-stage decision rule for testing genetic pleiotropy. Our proposed methodology is then applied to a set of data concerning tropical storms.
Title:智能大数据时代的统计学发展
Speaker:李勇 (成都信息工程大学)
Abstract: 我们正在从消费互联网全面进入产业互联网时代,由德国和美国发起的工业4.0革命,我国在智能大数据新时代应该如何抓住这一机遇和挑战?作为以数据为研究对象的统计学科,在智能大数据的新时期,如何顺势而上,完成自己的历史使命?报告从智能大数据呈现的时代特征,智能大数据时代的统计学现状分析,以及智能大数据时代的统计学能力需求等角度进行论述。
Title: Non-Hermitian matrix-valued Brownian motion
Speaker:刘党政 (中国科学技术大学)
Abstract: Consider autocorrelation functions of characteristic polynomials for matrix-valued Brownian motion, whose entries are i.i.d. Brownian motions. We obtain exact duality formulae for certain observables and further investigate possible phase transition and critical phenomena. This talk is based on joint work with Lu Zhang.
Title: Estimation in functional single-index varying coefficient model
Speaker:冯三营 (郑州大学)
Abstract: Functional regression allows for a scalar response to be dependent on a functional predictor, however, not much work has been done when scalar predictors that interacts with the functional predictor are introduced. In this paper, we introduce a new functional single-index varying coefficient model with the functional predictor being single-index part. By means of functional principal components analysis and basis function approximation, we obtain the estimators of slope function and coefficient functions, and propose an iterative estimating procedure. Furthermore, the rates of convergence of the proposed estimators and the mean squared prediction error are established under some regularity conditions. At last, we illustrate the finite sample performance of our proposed methods with some simulation studies and a real data application.
Title:Lassoing Eigenvalues
Speaker:伊梦茜 (对外经济贸易大学)
Abstract: The properties of penalized sample covariance matrices depend on the choice of the penalty function. In this talk, I will introduce a class of nonsmooth penalty functions for the sample covariance matrix and demonstrate how their use results in a grouping of the estimated eigenvalues. We refer to the proposed method as lassoing eigenvalues, or the elasso.
Title:列正交强正交表的构造
Speaker:刘民千 (南开大学)
Abstract: 强正交表是国际上新近提出的用于计算机试验的一类空间填充设计,其在低维空间填充性方面的表现要优于传统的正交表。为了使设计具有列正交性并改进其低维空间填充性,我们提出并构造了一类新的列正交强正交表,并研究了新设计的优良性质。所构造的强正交表水平数有所增加,同时在一维和二维的空间填充性有所增强。与现有文献中的结果相比,所构造设计可以容纳同等甚至更多因子,具有灵活的试验次数和列正交性。设计的构造方法简单灵活,所得设计是计算机试验的优良选择。
Title:Some applications of Median-of-Means method
Speaker:刘鹏飞 (江苏师范大学)
Abstract: We use the median-of-means(MoM)method to estimate the parameters of some statistical model and prove the consistency and asymptotic normality of the MoM estimator. Especially when there are outliers, the MoM estimator is more robust than other estimators. And on this basis, we propose hypothesis testing statistics for the parameters using empirical likelihood. The simulation performance show the superiority of MoM estimator and tests statistic. We also apply the MoM method to analyze some real data sets.
Title: L1正则下的自适应P-样条估计与节点自动选择
Speaker: 李哲源(河南大学)
Abstract: 惩罚样条一直在函数的非参数估计中扮演重要角色,然而如何放置节点、如何构造平滑度可变的自适应估计,却一直未能得到有效解决。基于B-样条的数学特性,我将提出一种新的基于L1范数的惩罚思路,说明并实证该方法可以实现节点选择,并生成混合次数的逐段多项式估计。
Title:Subgroup-adaptive randomization for subgroup confirmation in clinical trials
Speaker:刘中强 (河南理工大学)
Abstract: A well-known issue when testing for treatment-by-subgroup interaction is its low power, as clinical trials are generally powered for establishing efficacy claims for the overall population, and they are usually not adequately powered for detecting interaction (Alosh, Huque, & Koch [2015] Journal of Biopharmaceutical Statistics, 25, 1161–1178). Hence, it is necessary to develop an adaptive design to improve the efficiency of detecting heterogeneous treatment effects within subgroups. Considering Neyman allocation can maximize the power of usual 𝑍-test (see p. 194 of the book edited by Rosenberger and Lachin), we propose a subgroup-adaptive randomization procedure aiming to achieve Neyman allocation in both predefined subgroups and overall study population in this paper. To verify whether the proposed randomization procedure works as intended, relevant theoretical results are derived and displayed. Numerical studies show that the proposed randomization procedure has obvious advantages in power of tests compared with complete randomization and Pocock and Simon’s minimization method.
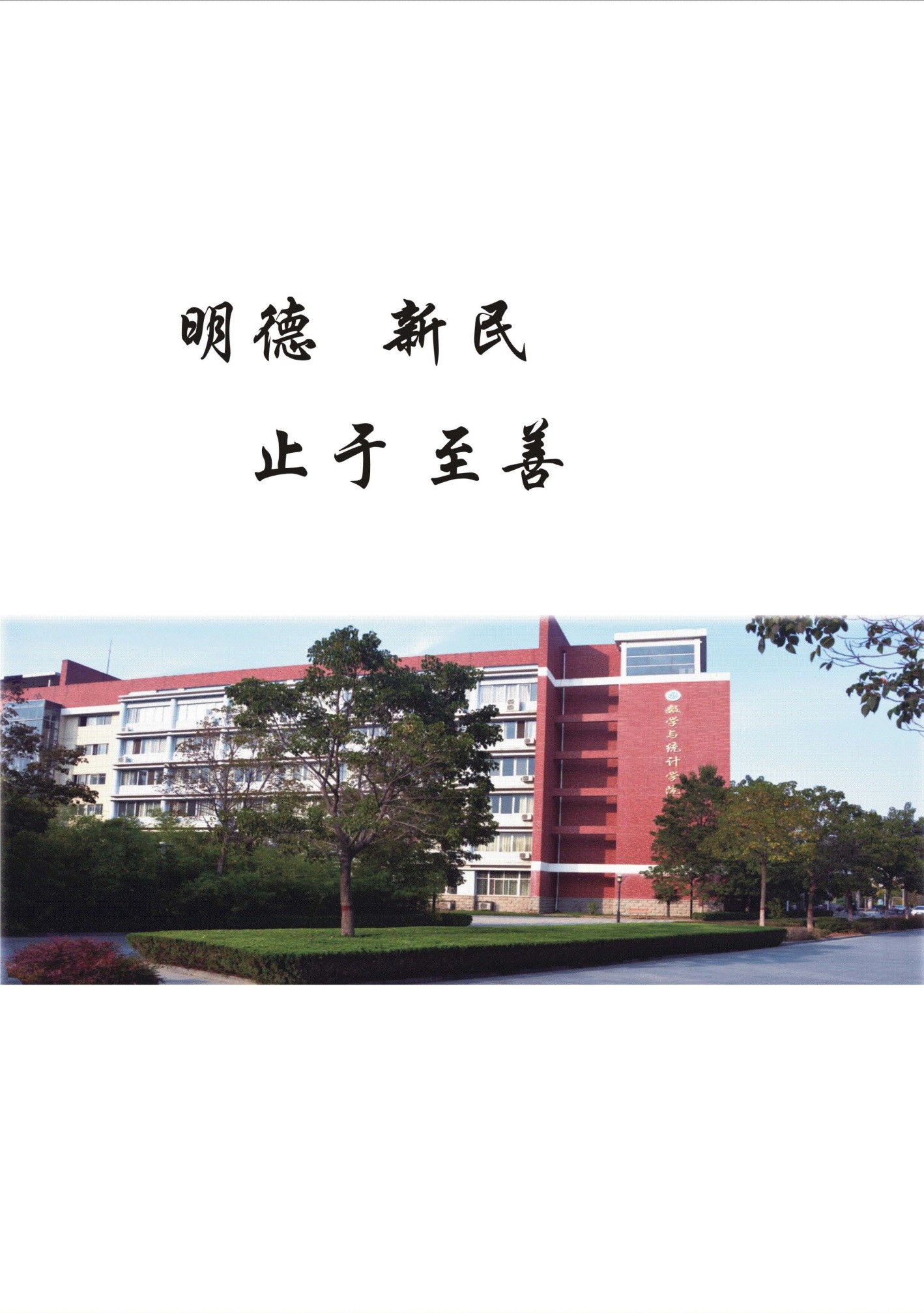