河南大学
2020年统计学前沿学术研讨会
暨博士后论坛
会议手册
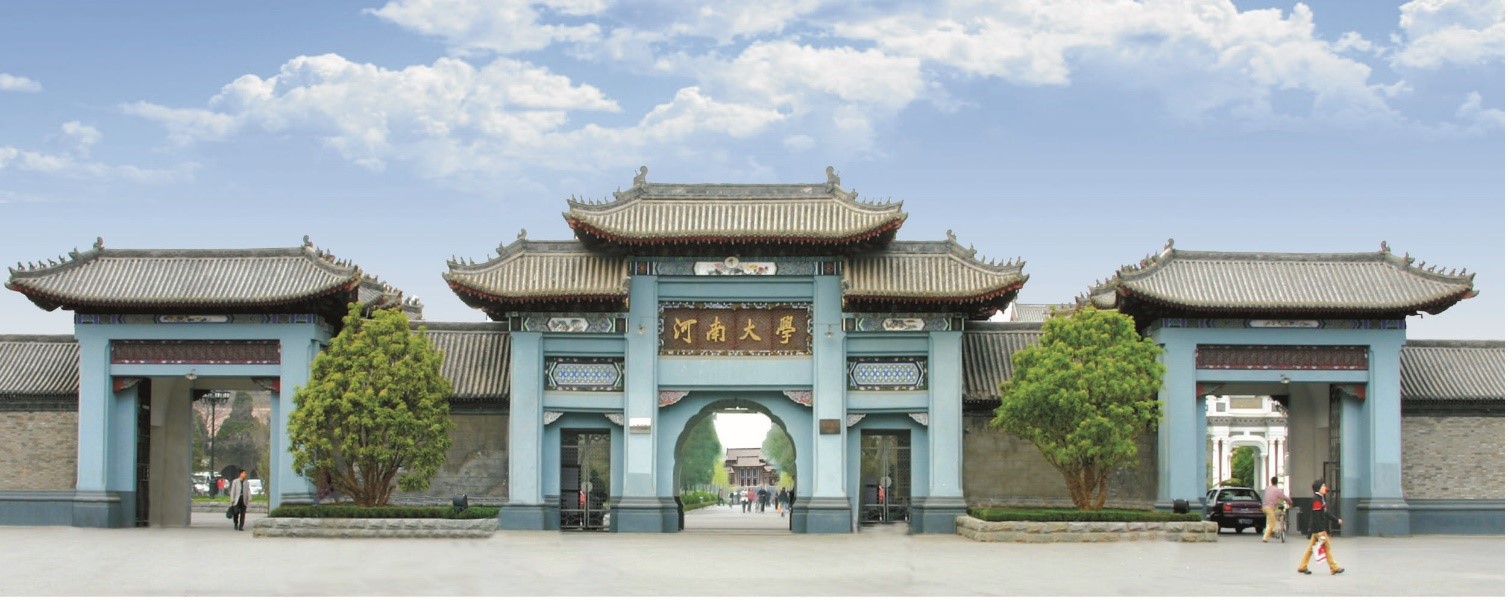
7月19日-7月20日
日程安排
2020年7月19日(星期日)上午,腾讯会议ID: 331 464 976 |
时间 |
报告人 |
报告题目 |
8:30-9:00 |
开幕式 |
主持人: 解俊山(河南大学) |
主持人: 薛留根(北京工业大学) |
9:00-9:35 |
朱文圣 (东北师范大学) |
Large-Scale Covariate Assisted Two-Sample Inference under Dependence |
9:40-10:15 |
张军舰 (广西师范大学) |
基于经验欧氏似然的变点检测 |
10:20-10:40 |
休息讨论 |
主持人:张军舰(广西师范大学) |
10:40-11:15 |
薛留根 (北京工业大学) |
Empirical likelihood for partially linear single-index models with missing observations |
11:20-11:55 |
刘 洋 (华东师范大学)
|
Maximum likelihood abundance estimation from capture–recapture data when covariates are missing at random |
2020年7月20日(星期一)上午, 腾讯会议ID: 615 890 549 |
主持人: 梁汉营(同济大学) |
8:30-9:05 |
邓 柯 (清华大学) |
Integrated Partition-Mallows Model and Its Inference for Rank Aggregation |
9:10-9:45 |
贾金柱 (北京大学) |
On the Efficiency of Logistic Regression Estimators in Estimating the Causal Effect |
9:50-10:10 |
休息讨论 |
主持人:王海斌(厦门大学) |
10:10-10:45 |
梁汉营 (同济大学) |
Quantile regression of partially linear single-index model with missing observations |
10:50-11:25 |
赵占平 (黄淮学院) |
2x2列联表中归因风险的统计推断 |
11:30-12:05 |
郑 晨 (河南大学) |
基于贝叶斯决策的遥感数据地物聚类分析 |
注: 每位老师报告结束后,预留5分钟讨论时间
题目与摘要
Title:Large-Scale Covariate Assisted Two-Sample Inference under Dependence
Speaker:朱文圣 (东北师范大学)
Abstract: The problems of large-scale two-sample inference often arise from the statistical analysis of “high throughput” data. The conventional multiple testing procedures for large-scale two-sample inference usually suffer from substantial loss of testing efficiency when conducting numerous two-sample t-tests directly. To some extent, this is due to the ignorance of sparsity information in large-scale two-sample inference. Moreover, in practice, the two-sample tests commonly have local correlations and neglecting the dependence structure in the two-sample tests may decrease the statistical accuracy in multiple testing. Therefore, it is imperative to develop a multiple testing procedure which can not only take into account the sparsity information but also accommodate the dependence structure among the tests. To address the aforementioned important issues, we first introduce a novel dependence model to allow for sparsity information and to characterize the dependence structure among the tests. Based on the dependence model, we propose a Covariate Assisted Local Index of Significance (COALIS) procedure and show that it is valid and optimal in some sense. Then a data-driven procedure is developed to mimic the oracle procedure and simulations show that COALIS procedures outperform their competitors. Finally, we apply COALIS procedure to the dosage response data.
Title:基于经验欧氏似然的变点检测
Speaker:张军舰 (广西师范大学)
Abstract: 变点问题一直是统计学中比较热门的一个研究方向,在实际生活中有广泛的应用。它针对一个序列数据, 在某一点前后的分布发生了变化,变点检测就是寻找数据分布发生变化的那一点, 同时考虑其相应的一些统计性质等。论文主要用经验欧氏似然方法研究均值模型以及线性模型中的变点问题,根据模型特点构造了截断经验欧氏似然比检验函数并给出显式表达;证明了零假设下检验统计量的极限分布为极值分布;利用该极限分布给出变点的诊断方法; 在有变点的情况下, 进一步给出变点位置的估计及其相合性的理论证明; 数值模拟、尼罗河年流量、黄石公园间歇性喷泉等实证分析表明了本文方法的有效性和实用性.
Title: Empirical likelihood for partially linear single-index models with missing observations
Speaker: 薛留根(北京工业大学)
Abstract: In this talk, we study the empirical likelihood for a partially linear single-index model with a subset of covariates and response missing at random. By using the bias-correction and the imputation method, two empirical log-likelihood ratios are proposed such that any of two ratios is asymptotically chi-squared. Two maximum empirical likelihood estimates of the index coefficients and the estimator of link function are constructed, their asymptotic distributions and optimal convergence rate are obtained. It is proved that our methods yield asymptotically equivalent estimators for the index coefficients. An important feature of our methods is their ability to handle missing response and/or partially missing covariates. In addition, we study the estimation and empirical likelihood for two special cases---the single-index model and partially linear model with observations are missing at random. A simulation study indicates that the proposed methods are comparable for bias and standard deviation, as well as in terms of coverage probabilities and average areas (lengths) of confidence regions (intervals). The proposed methods are illustrated by an example of real data.
Title:Maximum likelihood abundance estimation from capture–recapture data when covariates are missing at random
Speaker:刘洋 (华东师范大学)
Abstract: In capture–recapture experiments, individual covariates may be subject to missingness, especially when the number of captures is small. When the covariate information is missing at random, the inverse probability weighting method and the multiple imputation method are widely used to obtain point estimators of the abundance. These estimators are then used to construct Wald–type confidence intervals. However, such intervals may have seriously inaccurate coverage probabilities. In this talk, we present a maximum empirical likelihood (EL) estimation approach for the abundance in the presence of missing covariates. We show that the maximum EL estimator is asymptotically normal, and that the EL ratio statistic for the abundance has a chisquare limiting distribution with one degree of freedom. Simulations indicate that the proposed estimator has a smaller mean square error than existing estimators, and the proposed EL ratio confidence interval usually has more accurate coverage probabilities than the existing Wald–type confidence intervals. We illustrate the proposed method by analyzing data collected in Hong Kong for the yellow–bellied prinia, a bird species.
Title:Integrated Partition-Mallows Model and Its Inference for Rank Aggregation
Speaker:邓柯 (清华大学)
Abstract: Learning how to rank and how to aggregate ranking lists has been an area of active research for many years and its advances have played a vital role in the recent boom of internet commerce. The problem of discerning reliability of rankers based only on the rank data is of great interest to many practitioners, but has received less attention from researchers. By dividing the ranked entities into relevant and irrelevant(background) groups and incorporating the Mallows model, we propose a framework that can not only distinguish quality differences among the rankers, but also provide the detailed ranking information for relevant entities. Advantages of the proposed approach in comparison with existing ones are demonstrated via simulation studies and real-data applications. Extensions of our method to handle partial ranking lists and conduct covariate-assisted rank aggregation are also discussed.
Title:On the Efficiency of Logistic Regression Estimators in Estimating the Causal Effect
Speaker:贾金柱 (北京大学)
Abstract: This talk is about the Logistic Regression Estimators of the Average Treatment Effect (ATE) in randomized experiments, when the potential outcomes are binary variables. Based on some regularity conditions, exact expressions of asymptotic variances of the estimators are provided which is considered to be an important criterion for evaluating asymptotic efficiency. Also, we’ll compare efficiency of different estimators of ATE (including the regression adjustment estimator) and prove some important results. We find that introducing interaction terms between the assignment variable and the covariate sometimes helps improve asymptotic efficiency. Numerical simulations are carried out to verify the theoretical results and a counterexample is given to show that sometimes introducing interaction terms in Logistic Regression might make the estimator of ATE less efficient.
Title:Quantile regression of partially linear single-index model with missing observations
Speaker:梁汉营 (同济大学)
Abstract: In this talk, we discuss the quantile regression and variable selection of partially linear single-index model when data are missing at random, which allows the response and covariates missing simultaneously. By using iteration algorithm and local linear method, we construct the inverse probability weighted quantile estimators of both the parameters and the link function. The penalized estimation of the parameters is also considered based on the adaptive LASSO penalty. The asymptotic distributions and the oracle property of the proposed estimators are derived. Simulation study and real data analysis are presented to show the performance of the proposed methods.
Title:2x2 列联表中归因风险的统计推断
Speaker:赵占平 (黄淮学院)
Abstract: 2x2列联表的统计推断在现代医学,各种临床试验及现代生物学的研究中通常是感性趣的问题,其中归因风险的统计推断经常出现在流行病学和健康服务研究中. 本文我们针对2x2列联表中归因风险的统计推断给出一种新的检验方法,利用2x2列联表中边际和条件概率提出了Score检验,给出了建立在置信区间之上的Score检验的描述,并对Score检验和似然比检验的效果依据覆盖概率、左尾错误概率及右尾错误概率进行评价,最终得出结论Score检验比似然比检验效果更有效.
Title:基于贝叶斯决策的遥感数据地物聚类分析
Speaker:郑晨 (河南大学)
Abstract: 遥感影像是一类重要的对地观测数据,广泛应用于资源、环境、灾害、区域、城市等方面调查、监测、分析和预测的工作。马尔科夫随机场模型(Markov random field,MRF)是一种概率图模型,因其有效的数据空间描述能力和完备的理论基础,在遥感影像地物聚类识别方面受到了广泛关注。但是,MRF模型的二阶势函数往往不能刻画不同地物类别间的相依关系,针对该问题,提出了一种基于贝叶斯决策的MRF模型聚类方法。该方法提出了一种各向异性的惩罚矩阵来表示不同地物类别间关系的差异性,并以此作为损失函数,MRF模型条件概率为后验分布,通过后验风险准则得到最终的聚类结果。纹理、航拍和高分2号影像数据的实验结果验证了本文方法的有效性。
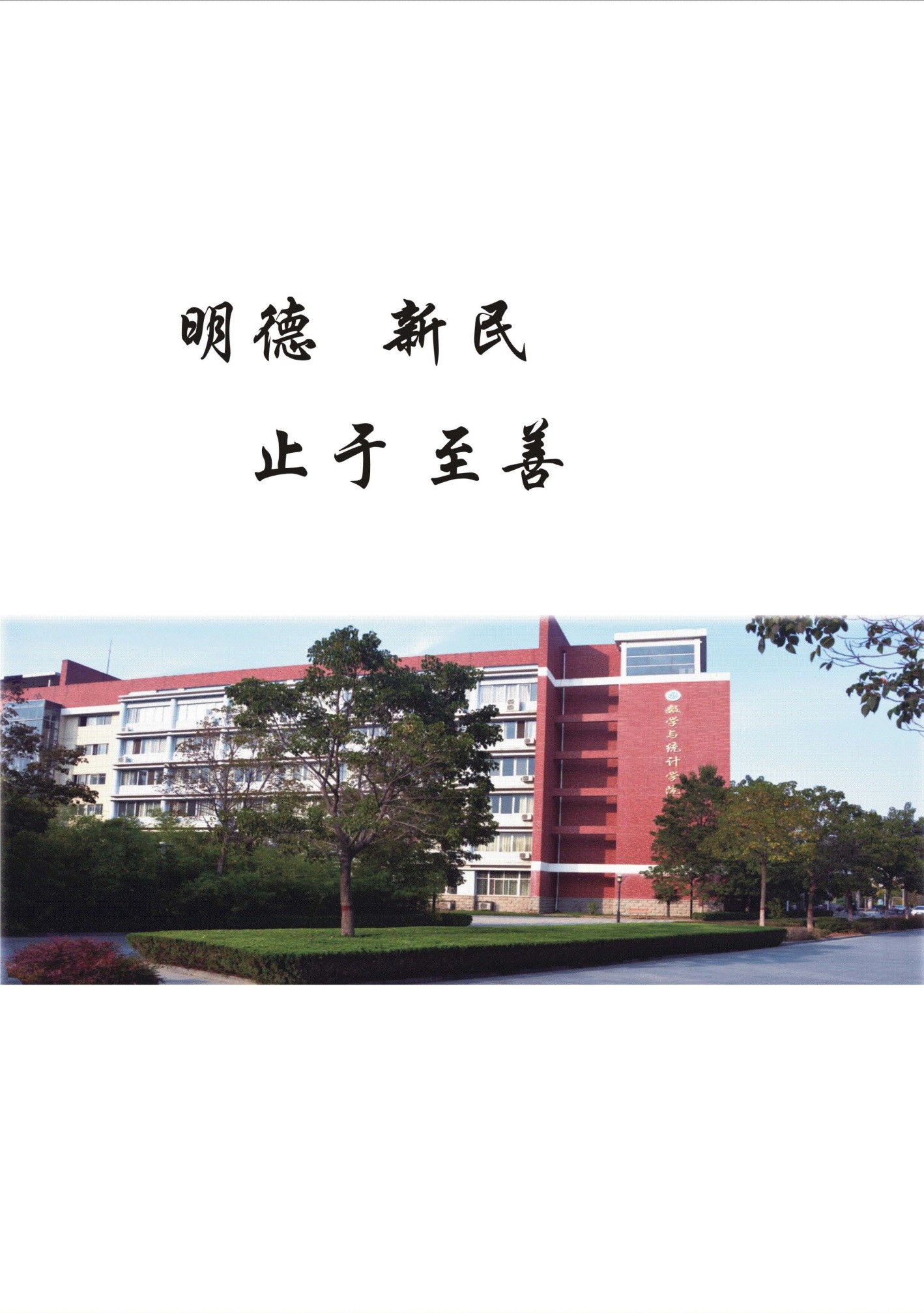